Python自动化炒股:利用LightGBM和CatBoost进行股票市场预测的详细指南

Python自动化炒股:利用LightGBM和CatBoost进行股票市场预测的详细指南
引言
在当今的金融市场中,数据驱动的决策变得越来越重要。Python作为一种强大的编程语言,为投资者提供了自动化炒股的工具。在这篇文章中,我们将探讨如何使用LightGBM和CatBoost这两种先进的机器学习算法来预测股票市场的走势。我们将从基础概念讲起,逐步深入到实际代码实现,帮助你构建自己的股票市场预测模型。
什么是LightGBM和CatBoost?
LightGBM
LightGBM是一种基于梯度提升框架的高效机器学习算法,由微软开发。它使用基于树的学习算法,特别适合处理大规模数据集,并且在速度和效率上都有显著优势。
CatBoost
CatBoost是另一种基于梯度提升的算法,由Yandex开发。它特别擅长处理分类特征(categorical features),无需手动转换为数值型数据,这在金融数据分析中非常有用。
准备工作
在开始之前,你需要安装一些Python库。如果你还没有安装,可以通过以下命令安装:
pip install numpy pandas scikit-learn lightgbm catboost
数据获取
我们将使用一个公开的股票市场数据集来演示。这里我们使用pandas
库来加载和处理数据。
import pandas as pd
# 加载数据集
data = pd.read_csv('stock_data.csv')
print(data.head())
数据预处理
在训练模型之前,我们需要对数据进行预处理。这包括处理缺失值、转换分类特征等。
# 处理缺失值
data.fillna(method='ffill', inplace=True)
# 转换分类特征
categorical_features = ['Industry', 'Sector']
data[categorical_features] = data[categorical_features].apply(lambda x: x.astype('category').cat.codes)
特征工程
特征工程是构建有效预测模型的关键步骤。我们需要选择合适的特征,并可能创建新的特征来提高模型的性能。
# 创建新特征:过去几天的收盘价平均值
for i in range(1, 6):
data[f'Close_Mean_{i}d'] = data['Close'].rolling(window=i).mean()
# 选择特征
features = data.drop(['Close'], axis=1)
target = data['Close']
划分数据集
我们将数据集划分为训练集和测试集,以便评估模型的性能。
from sklearn.model_selection import trAIn_test_split
X_train, X_test, y_train, y_test = train_test_split(features, target, test_size=0.2, random_state=42)
训练LightGBM模型
现在我们可以开始训练LightGBM模型了。
import lightgbm as lgb
# 创建LightGBM数据格式
lgb_train = lgb.Dataset(X_train, y_train)
lgb_eval = lgb.Dataset(X_test, y_test, reference=lgb_train)
# 设置参数
params = {
'boosting_type': 'gbdt',
'objective': 'regression',
'metric': {'l2', 'l1'},
'num_leaves': 31,
'learning_rate': 0.05,
'feature_fraction': 0.9,
}
# 训练模型
gbm = lgb.train(params,
lgb_train,
num_boost_round=20,
valid_sets=lgb_eval,
early_stopping_rounds=5)
# 预测
predictions_lgbm = gbm.predict(X_test)
训练CatBoost模型
接下来,我们训练CatBoost模型。
from catboost import CatBoostRegressor
# 创建CatBoost模型
model = CatBoostRegressor(iterations=100,
learning_rate=0.1,
depth=6,
loss_function='RMSE',
verbose=200)
# 训练模型
model.fit(X_train, y_train, eval_set=(X_test, y_test), use_best_model=True)
# 预测
predictions_catboost = model.predict(X_test)
模型评估
我们使用均方误差(MSE)来评估模型的性能。
from sklearn.metrics import mean_squared_error
mse_lgbm = mean_squared_error(y_test, predictions_lgbm)
mse_catboost = mean_squared_error(y_test, predictions_catboost)
print(f"LightGBM MSE: {mse_lgbm}")
print(f"CatBoost MSE: {mse_catboost}")
结论
通过这篇文章,我们学习了如何使用LightGBM和CatBoost来预测股票市场。这两种算法各有优势,可以根据具体问题选择合适的模型。记住,模型的性能很大程度上取决于数据的
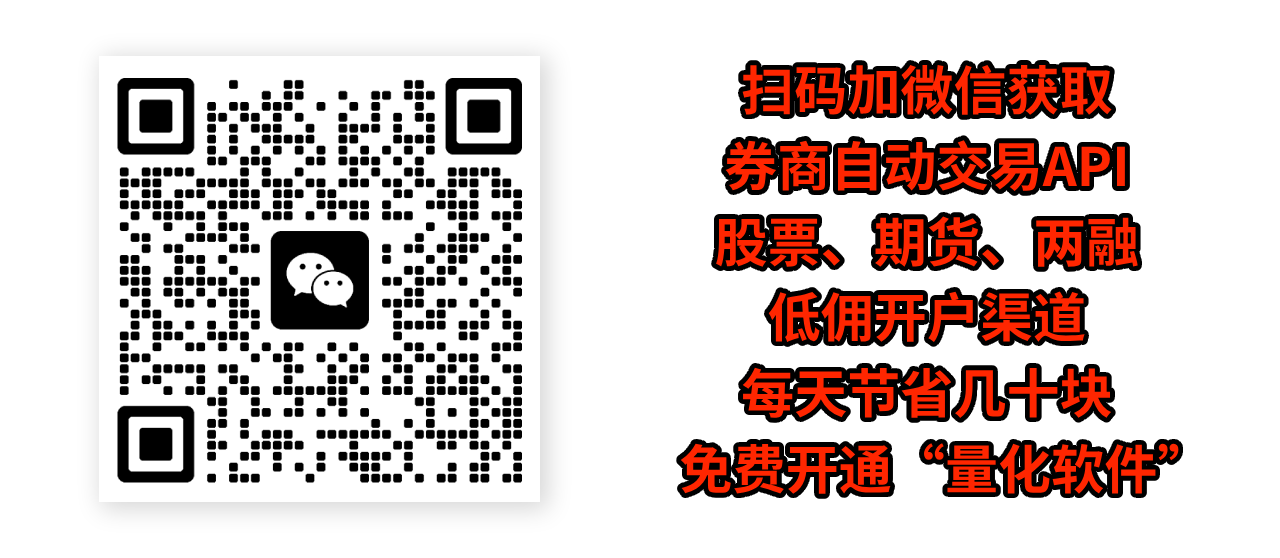
探讨名词“独特并购范式”的真正意义
« 上一篇
2024-11-03
名词“独特指数逻辑”体现了哪些核心理念?
下一篇 »
2024-11-03