如何利用Python进行股票市场的智能材料分析?
如何利用Python进行股票市场的智能材料分析?
在当今快速发展的股票市场中,投资者和分析师们不断地寻找新的方法来分析和预测股票价格。智能材料分析(Intelligent Material Analysis)是一种新兴的方法,它通过结合机器学习、自然语言处理和数据挖掘技术,来提取和分析股票市场中的关键信息。本文将介绍如何利用Python进行股票市场的智能材料分析,帮助投资者更好地理解市场动态并做出明智的投资决策。
1. 数据收集
在进行智能材料分析之前,首先需要收集相关的股票数据。Python中有许多库可以帮助我们获取股票数据,例如yfinance
和pandas-datareader
。
import yfinance as yf
# 获取苹果公司的股票数据
data = yf.download('AAPL', start='2020-01-01', end='2023-01-01')
2. 数据预处理
数据预处理是智能材料分析中的关键步骤,它包括数据清洗、特征工程等。
import pandas as pd
# 清洗数据,去除缺失值
data.dropna(inplace=True)
# 特征工程,计算移动平均线
data['SMA_50'] = data['Close'].rolling(window=50).mean()
data['SMA_200'] = data['Close'].rolling(window=200).mean()
3. 自然语言处理(NLP)
自然语言处理是智能材料分析中的重要组成部分,它可以帮助我们从新闻、社交媒体等非结构化数据中提取有价值的信息。
from nltk.corpus import stopwords
from nltk.tokenize import word_tokenize
import nltk
# 下载NLP资源
nltk.download('punkt')
nltk.download('stopwords')
# 定义一个函数来清洗文本
def clean_text(text):
stop_words = set(stopwords.words('english'))
words = word_tokenize(text)
filtered_words = [word for word in words if word.isalpha() and word not in stop_words]
return ' '.join(filtered_words)
# 假设我们有一个新闻标题列表
news_titles = ['Apple launches new iPhone', 'Tech stocks rise']
# 清洗新闻标题
cleaned_titles = [clean_text(title) for title in news_titles]
4. 情感分析
情感分析可以帮助我们理解市场对特定事件或新闻的反应。我们可以使用TextBlob
库来进行情感分析。
from textblob import TextBlob
# 对清洗后的新闻标题进行情感分析
for title in cleaned_titles:
blob = TextBlob(title)
print(blob.sentiment)
5. 机器学习模型
在智能材料分析中,机器学习模型可以用来预测股票价格或市场趋势。我们可以使用scikit-learn
库来构建一个简单的线性回归模型。
from sklearn.linear_model import LinearRegression
from sklearn.model_selection import trAIn_test_split
# 选择特征和目标变量
X = data[['SMA_50', 'SMA_200']]
y = data['Close']
# 划分训练集和测试集
X_train, X_test, y_train, y_test = train_test_split(X, y, test_size=0.2, random_state=42)
# 构建线性回归模型
model = LinearRegression()
model.fit(X_train, y_train)
# 预测测试集
predictions = model.predict(X_test)
6. 结果评估
评估模型的性能是智能材料分析中不可或缺的一步。我们可以使用mean_squared_error
来评估模型的预测准确性。
from sklearn.metrics import mean_squared_error
# 计算均方误差
mse = mean_squared_error(y_test, predictions)
print(f'Mean Squared Error: {mse}')
7. 可视化
最后,我们可以使用matplotlib
库来可视化模型的预测结果,以便更好地理解模型性能。
import matplotlib.pyplot as plt
# 绘制实际值和预测值
plt.figure(figsize=(10, 6))
plt.plot(y_test.index, y_test, label='Actual')
plt.plot(y_test.index, predictions, label='Predicted')
plt.title('Stock Price Prediction')
plt.xlabel('Date')
plt.ylabel('Price')
plt.legend()
plt.show()
结论
通过上述步骤,我们可以看到如何利用Python进行股票市场的智能材料分析。这种方法结合了数据科学、自然语言处理和机器学习技术,为投资者提供了一种全新的视角来分析和预测股票市场。随着技术的不断进步,智能材料分析将在股票市场中扮演越来越重要的角色。
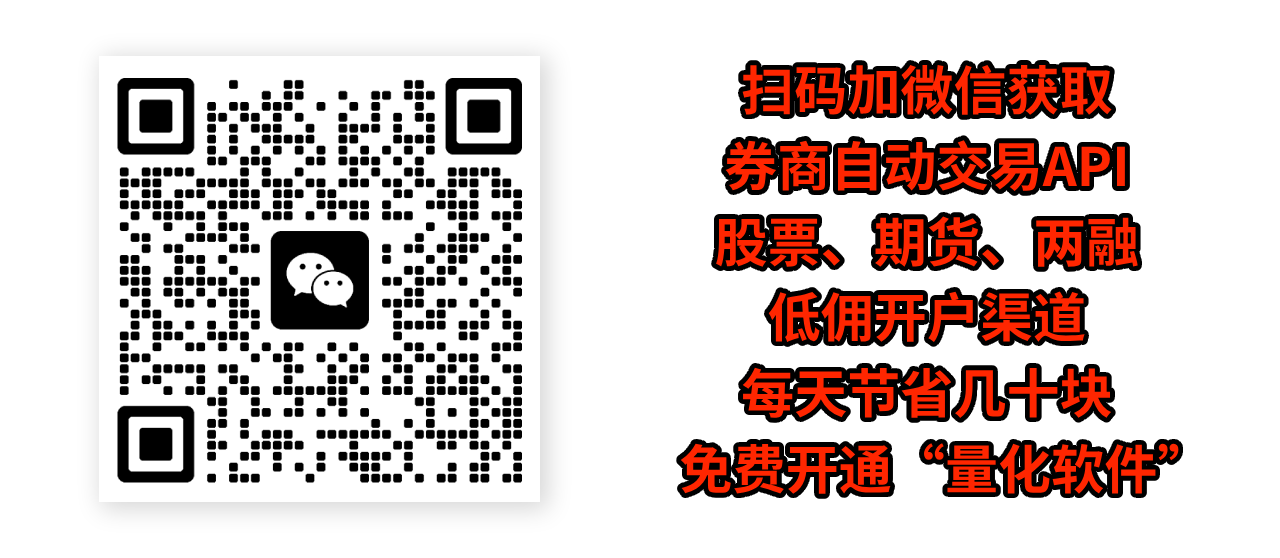
一起探讨:名词“爆发炒股产品”的定义与作用
« 上一篇
2024-09-30
深入研究:什么是名词“爆发期货平台”?
下一篇 »
2024-09-30